Handling Data with Many Labels Using Neural Networks
As time and space complexities grow linearly as the label size increases, it is inefficient to train models in its original label space. We consider adopting AttentionXML [YZW+19] to address the issue by training models with a reduced space of labels.
Usage
It is recommended to run AttentionXML through a configuration file. For example, to test AttentionXML on Wiki10-31K, run the following code:
$ python main.py -c example_config/Wiki10-31K/attentionxml.yml
Training Process
Roughly speaking, training AttentionXML takes two steps. First, a model (model 0) is trained to predict clusters (rather than labels). Then, another model (model 1) is trained to predict labels. Both models involve a BiLSTM layer with label-wise attention.
One significant distinction between AttentionXML and one-vs-all algorithms is that in training model 1, AttentionXML only updates weights related to only a subset of the original label space (in the attention layer) during backpropagation, thereby increasing training speed.
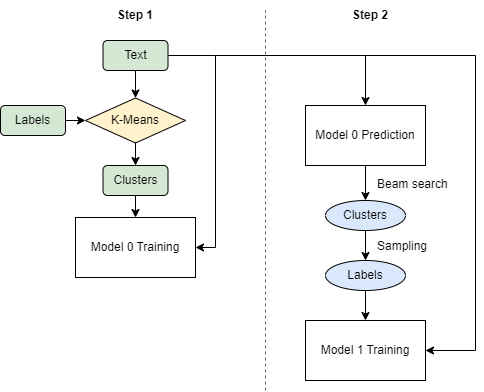
Hyperparameters
There are 2 extra hyperparameters for AttentionXML that users need to know:
cluster_size: The maximal number of labels in a cluster.
beam_width: The process of selecting predicted clusters from model 0 is called beam search. Beam width indicates the number of clusters that will be selected.
Performance
We compared the performance between BiLSTM and AttentionXML as they have similar architectures. The dataset, Wiki10-31K, has 30,938 classes, which makes it hard for models to train in a one-vs-all manner.
Both models were trained on an A100 Nvidia GPU. Their test results are shown below. Notice the difference between their running time.
Performance |
P@1 |
P@3 |
P@5 |
Time (min) |
---|---|---|---|---|
BiLSTM |
84.48 |
75.91 |
66.88 |
87.1 |
AttentionXML |
87.44 |
77.70 |
67.85 |
29.9 |